The Sequential Quadratic Programming Solver |
Conditions of Optimality
To facilitate discussion of the optimality conditions, we present the notation to be used for easy reference:

- number of general nonlinear constraints, which include the linear
constraints but not the bound constraints

- dimension of
, i.e., the number of decision variables

- iterate, i.e., the vector of
decision variables

- objective function

- gradient of the objective function

- Hessian matrix of the objective function

- Lagrange multiplier vector,

- Lagrangian function of constrained problems

- gradient of the Lagrangian function with
respect to

For

and

, the superscript

is used to denote the value at the

th iteration, such as

, and the superscript

is used to denote their corresponding optimal values, such as

.
We rewrite the general form of
nonlinear optimization problems in the section "Overview" by grouping the equality
constraints and inequality constraints. We also rewrite all the general nonlinear
inequality constraints and bound constraints in one form as ``
'' inequality
constraints. Thus we have the following:
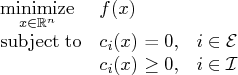
where

is the set of indices of the equality constraints,

is the set of
indices of the inequality constraints, and

.
A point
is feasible if it satisfies all the constraints
, and
.
The feasible region
consists of all the feasible points. In unconstrained
cases, the feasible region
is the entire
space.
A feasible point
is a local solution of the problem if there exists a
neighborhood
of
such that

Further, a feasible point

is a
strict local solution if strict inequality
holds in the preceding case, i.e.,

A feasible point

is a
global solution of the problem if no point in

has a smaller function value than

), i.e.,

The SQP solver finds a local minimum of an optimization problem.
The following conditions hold for unconstrained optimization problems:
- First-order necessary conditions: If
is a local solution and
is
continuously differentiable in some neighborhood of
, then

- Second-order necessary conditions: If
is a local solution and
is twice continuously differentiable in some neighborhood of
, then
is positive semidefinite.
- Second-order sufficient conditions: If
is twice continuously differentiable in some neighborhood of
, and
if
and
is positive definite, then
is a
strict local solution.
For constrained optimization problems, the
Lagrangian function is defined as
follows:

where

, are called
Lagrange multipliers.

is used to denote the gradient of the Lagrangian function
with respect to

, and

is used to denote the Hessian of the
Lagrangian function with respect to

. The
active set at a feasible point

is
defined as

We also need the following definition before we can state the first-order and second-order necessary conditions:
- Linear independence constraint qualification and regular point: A point
is
said to satisfy the linear independence constraint qualification if the gradients of active constraints

are linearly independent. We refer to such a point
as a regular point .
We now state the theorems that are essential in the analysis and design of
algorithms for constrained optimization:
- First-order necessary conditions: Suppose
is a local minimum and also a regular point. If
and
, are
continuously differentiable, there exist Lagrange multipliers
such that the following conditions hold:
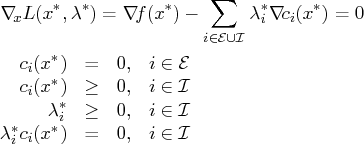
The preceding conditions are often known as the Karush-Kuhn-Tucker conditions , or KKT conditions for short.
- Second-order necessary conditions: Suppose
is a local minimum and also a regular point. Let
be the Lagrange multipliers that
satisfy the KKT conditions. If
and
, are twice
continuously differentiable, the following conditions hold:

for all
that satisfy

- Second-order sufficient conditions: Suppose there exist a point
and some
Lagrange multipliers
such that the KKT conditions are satisfied. If
the conditions

hold for all
that satisfy

then
is a strict local solution.
Note that the set of all such
's forms the null space of matrix
. Hence we can numerically check
the Hessian of the Lagrangian function projected onto the null space. For a rigorous
treatment of the optimality conditions, see Fletcher (1987) and
Nocedal and Wright (1999).
The SQP solver declares a solution to be optimal when all the following criteria are satisfied:
The last four points imply that the complementarity error, defined as
,
, approaches zero as the solver progresses.
The SQP solver provides the HESCHECK option to verify that the second-order necessary conditions are also satisfied.